GLM Pricing
GLM pricing, or Generalised Linear Model pricing, is a statistical method used in the insurance industry to determine the pricing of insurance policies. It involves using generalised linear models to analyse and predict the expected cost of claims based on various risk factors associated with the insured entities. These models enable actuaries and analysts to incorporate different types of data and relationships between variables, such as the linear or exponential relationship between risk factors and claim costs, thereby providing a more sophisticated and accurate pricing mechanism than simpler linear models. This method is particularly valued for its ability to handle skewed and non-normal data, which is common in insurance claims.
Introduction to Generalised linear models (GLMs)
Generalised linear models (GLMs) are a means of modelling the relationship between a variable whose outcome we wish to predict and one or more explanatory variables. The approach of using GLMs to set prices is well-established and standardised. GLMs have been widely used as the main actuarial pricing technique for pricing actuaries in the insurance industry for more than a decade in the UK.
Generalised linear modelling identifies statistically significant relationships between a set of risk factors (explanatory variables) which can then be used to produce an estimate of the response variable. In insurance pricing, GLMs are typically used to produce an estimate of the expected value of
- Claim frequency (i.e., claims per exposure)
- Claim severity (i.e., of loss per claim)
- Pure premium (i.e., loss per exposure)
- Loss ratio (i.e., loss per pound of premium)
Once the expected loss has been estimated, insurance companies can combine this with rates for various risk factors to set premiums that should cover anticipated claims, allowing their company a profit, and keeping them competitive in the marketplace.
If inaccurate premiums are set, it could result in solvency issues for the insurance companies or cause them to be uncompetitive in the market. According to Accenture, getting the best price is the top priority for 52% of auto insurance customers, 50% of home insurance customers and 38% of life insurance customers. So, it is vital for the insurance company and policyholder that the GLM used accurately predicts the expected loss and accurate premiums are set as a result.
Fundamentals of GLM Pricing: Understanding the Basics
A generalised linear model consists of three components: a random component, a systematic component, and a link function.
Random component
Each component of Y is independent and is from one of the exponential family of distributions.
Systematic component
A linear combination of the estimated parameters (βi) gives the linear predictor, η:
𝜂 = 𝑋. β
Link function
“Links” the relationships between the random and systematic components such that:
𝐸[𝑌] = 𝜇 = 𝑔 −1 (𝜂)
(Note that without the link function, the expression would just be simple linear regression.)
Applying this to an insurance context, the random component represents the response variable which could be something like the amount of the claim, which is assumed to follow a specific probability distribution from the exponential family.
The systematic component represents the predictors or independent variables that affect the likelihood of a claim, such as age, gender, location, and other risk factors. Estimation of the linear predictor parameters βi is by maximum likelihood.
The link function connects the random and systematic components and transforms the response variable into a linear form, which can be modelled using traditional linear regression techniques.
Data plays a crucial role as it forms the foundation for the model. Data is used in estimating the model parameters, making predictions, and assessing the quality of the model. Without quality data, the quality of the model would be severely limited. So, data should be thoroughly cleaned before being used in a GLM.
How Is Generalised Linear Modelling Applied in Insurance Pricing?
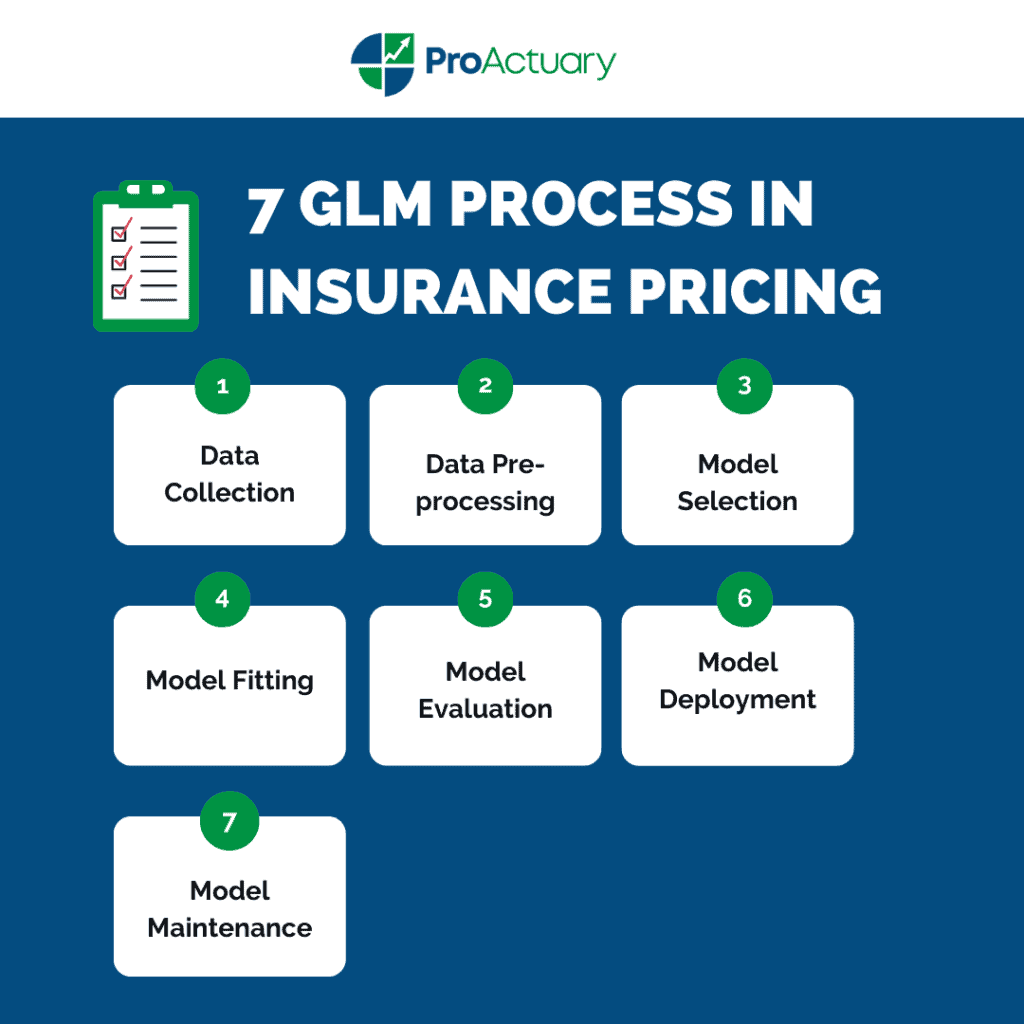
An iterative process would be used with the following steps:
- Data Collection: Collecting high-quality data is crucial for building accurate GLM models. This involves identifying relevant risk characteristics and claims experience data for policyholders.
- Data Pre-processing: Data pre-processing involves cleaning and transforming the collected data. This may involve removing missing or irrelevant data and transforming variables to fit the GLM assumptions.
- Model Selection: Selecting the distribution is important to accurately model the relationship between risk characteristics and claims experience. Common distributions used in insurance pricing models are from the exponential family (Poisson, exponential, gamma, normal, binomial). The “canonical” link corresponding to each response distribution can then be identified. Explanatory variables can then be chosen based on the rating factors for that product. For example, in car insurance factors such as vehicle type, driving history or years of driving experience may be used.
- Model Fitting: This step involves estimating the model parameters using the collected data. The fitting is usually done using software such as SAS, which implements maximum likelihood estimation or its variants.
- Model Evaluation: Once the model is fitted, it is important to evaluate its performance. This can involve calculating measures such as the Akaike Information Criterion (AIC) or Bayesian Information Criterion (BIC).
- Model Deployment: Once the model is evaluated, it can be used to estimate premiums for new policies based on the risk characteristics of the policyholder.
- Model Maintenance: Finally, it is important to continually monitor and update the GLM model to ensure it remains accurate and relevant over time.
GLM Pricing vs Traditional Pricing Methods
GLMs extend traditional linear regression models by allowing for more flexible distributions of the response variable, such as skewed or binary data, and incorporating additional explanatory variables.
Traditional methods include:
Experience rating (or burning cost): This method involves analysing an individual or group’s past loss experience to determine the cost of insurance premiums in the future. Insurers use this method to adjust premiums based on the policyholder’s history of claims.
Frequency-Severity: The method takes historical data to estimate the average number of claims and the overall average cost of each claim. Regarding frequency, the insurer is effectively anticipating the number of claims over a given period of time. If the frequency is high, it is indicative that a large number of claims is expected to occur.
Manual Rating: This method uses predetermined rates based on the type of coverage being provided, without considering individual or group characteristics. Manual rating is often used for small businesses and other types of policies where individual risk factors are difficult to assess.
Judgment Rating: This method involves the use of underwriters to assess an individual’s risk and determine an appropriate premium based on their experience and expertise. Judgment rating is often used for high-risk policies, such as commercial liability insurance.
Current Uses in the Market: Risk-Based Pricing and Price Optimisation
Risk-Based Pricing
A pricing method that involves setting prices based on the risk level of the customer or transaction. Under this approach, customers who are deemed to be at higher risk are charged higher premiums or fees to reflect the increased likelihood of loss from a claim. The less likely a customer is to make a claim, and the lower the value of that claim, the lower their premium will be. There is therefore a financial incentive for customers to reduce their risks.
Examples of risk-based pricing in different types of insurance:
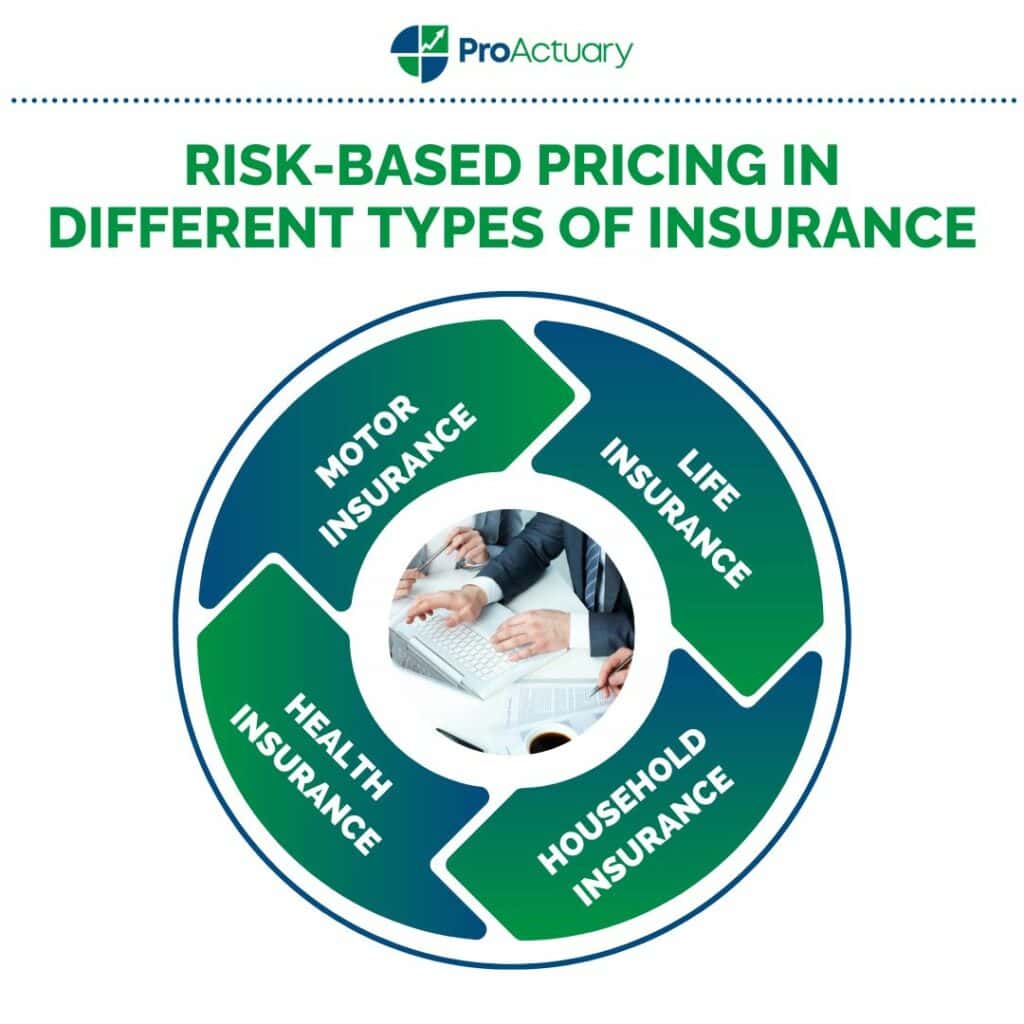
Motor Insurance
Insurers use a range of measures to encourage motorists to drive more safely. For example, lower premiums are usually available for drivers of cars with smaller engines or security systems, and discounts for drivers who complete additional training courses are common. These discounts are successful in encouraging motorists to think more carefully about safety – over 50% of drivers who complete the UK’s Pass Plus training course, which teaches new drivers about a wide range of hazards, do so because of the insurance discounts.
There is evidence that risk pricing encourages safer driving. In Canada, two different systems of motor insurance operate. While three provinces allow risk pricing, the other seven have pricing restrictions which means that everyone pays the same basic insurance premium. In the provinces where risk pricing is allowed, the chance of being involved in a fatal road crash is almost 20% lower than in the more heavily regulated provinces.
Life Insurance
Activities like smoking significantly increase the risk of premature death. 114,000 people in the UK die from smoking-related conditions every year, accounting for one-fifth of all UK deaths. Because of their greater health risks, smokers frequently pay up to twice as much for their life insurance cover compared to non-smokers. Over 30 years, a smoker will generally pay up to £6,000 more than a non-smoker on a £100,000 life insurance policy.
Health Insurance
Health insurers often reward customers for leading a healthy lifestyle. For example, Vitality’s private medical insurance offers a free Apple Watch Series 8 for those who average 12,500 steps per day over the course of their cover.
Household Insurance
While four-fifths of the public have window locks fitted in their homes, only one-third of burglary victims do. The more secure the property, the less likely it is to be burgled. Many insurers offer lower buildings and contents premiums to homeowners with window locks and burglar alarms, in recognition of the reduced crime risk associated with a more secure property. In some cases, insurers make these security devices a condition of cover.
Price Optimisation
Any movement away from a “technical” price is price optimisation. Essentially price optimisation is the use of customer demand to set prices. The insurer separates the ‘risk’ component from the ‘demand and elasticity’ component of a policy.
The first step is to calculate the underlying cost of providing the insurance policy i.e., “the technical price”. The insurer would then use a GLM to model the demand, or probability of sale as a function of different factors that influence demand using historical quote data for both customers who go on to buy and those who don’t. Randomised price trials can be used to model the demand elasticity, the change in demand at different prices.
An insurer’s pricing team can gain a granular understanding of both the profit per policy sold (from risk component modelling) and how the probability of purchase (from demand and elasticity components) varies as the premium changes. These components can then be combined to produce the expected profit for different premiums. The insurer would then choose a premium which allows them to make a suitable profit and stay competitive in the market.
Price optimisation is commonly used in general insurance. It is particularly effective in the car insurance market as there are lots of rating factors to be considered and it’s a very competitive market therefore rapid repricing to meet the real-time market price.
In life insurance, the majority of business is sold through financial advisers with business typically sold on guaranteed premiums with terms of 20-30 years. Providers quote ‘standard prices’ based on a low number of rating factors – typically sum assured, age, term, and smoker status. This lower availability of data means that it is more difficult to implement as rich a price optimisation model in life insurance. Despite its additional challenges, in recent years life insurance firms have started to utilise price optimisation.
The 2 current market applications demonstrate the benefits of using generalised linear modelling in the industry at the moment. They are flexible, shown by the fact they can incorporate a wide range of data including binary, continuous and categorical data. GLMs are efficient and can be run quickly which is vital for price optimisation in products such as car insurance, due to the rise of price comparison websites allowing customers to shop around for the best price. As generalised linear modelling is widely adopted in industry, insurers are clearly confident they can produce accurate prices.
Challenges and Limitations in GLM Pricing in Insurance Pricing
There is some market evidence suggesting that the GLMs pricing method is not always effective. For instance, despite using a comparable GLM methodology, the quoted premiums produced by models vary greatly amongst insurance firms. Even for a typical risk, the quoted premium could range from £200 to £1000+.
The IFOA has shown that GLMs might underestimate premiums in segments where the model is highly uncertain. GLMs also tend to push premiums towards an extreme level, which creates downside risk in a competitive market.
When using a GLM, either zero or full credibility is given to the data. When choosing factors in the GLM, the standard practice is to test all available factors in the data by fitting a factor into the model to decide whether such a factor is statistically significant or not. If it is, the factor will be kept in the model; otherwise, the factor will be excluded from the model. In making this decision, a significant level is usually arbitrarily chosen.
When a factor is considered as significant and kept in the model, full credibility will be given to the estimated parameter of that factor. However, the confidence level could never be 100%. Even if a confidence level of 99% is chosen, statistical significance means that there is still a 1% chance that the factor is the same as the base level. So, the estimated parameter should not be given full credibility.
On the other hand, if a factor does not pass the significance test, the estimated parameter still contains some information in the data and therefore should not be discarded completely from the model. This could happen when modelling a small segment, for example left-hand drive, or factors with many levels, for example postcode, vehicle type and occupation, as the limited volume reduces the significance of the results.
A potential solution is to use a Generalised Linear Mixed Model (GLMM) which could reduce the volatility of prediction. GLMM is an extension of GLM to a mixed model. The key difference between them is a random effect component in the linear predictor h for GLMM.
For further reading on the drawbacks of generalised linear models in insurance check out this 2013 article published by the IFOA: https://www.actuaries.org.uk/system/files/documents/pdf/c6-paper.pdf
Concluding Thoughts on GLM Pricing in Insurance
In conclusion, the use of Generalised Linear Models (GLMs) has proven to be a valuable tool for insurance pricing. By incorporating various factors and risk characteristics, GLMs have enabled insurance companies to price their policies more accurately, resulting in fairer premiums for policyholders and improved profitability for insurers. However, it is important to note the limitations of GLMs and they should be regularly reviewed and updated to ensure their continued accuracy and relevance. Provided they are used correctly, the use of GLMs in insurance pricing is a win-win scenario for both the policyholder and the insurer.
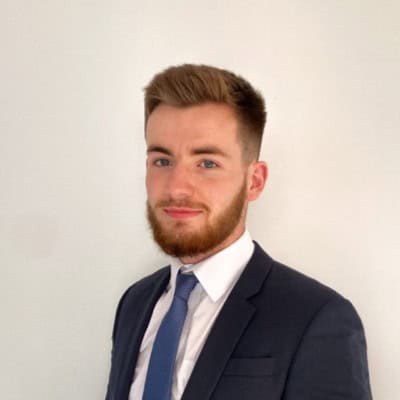
Kealan Friel
“Kealan Friel is an Equity Research Analyst at The Spark. Prior to that he was an Actuarial Intern at Allianz Ireland. Kealan graduated from Queen’s University Belfast in 2023 with a BSc degree in Actuarial Science and Risk Management. You can connect with him on LinkedIn.”