Will Artificial Intelligence Replace Actuaries?
Will Artificial Intelligence replace actuaries?
The answer to this question largely depends on the definition used for an “actuary”.
If you define an actuary as a professional who uses his/her expertise for evaluating and managing the financial impact of a multitude of differing risks, then developments in artificial intelligence can only make us more efficient at our duties.
In the simplest of terms, artificial intelligence (AI) techniques cannot just implement themselves and replace entire careers.
Somebody has to think of how to formulate these models, how to adapt and incorporate them into current working environments, how to maintain these models, and most importantly, how to interpret their results (a result in itself amounts to nothing if you cannot understand its meaning and significance to the wider organisation).
Who else could perform such duties within the actuarial industry other than actuaries themselves? So, for the short answer to this question, it is very hard to believe that advances in artificial intelligence will replace actuaries any time soon.
Now for a slightly more in-depth analysis…
Brief Thoughts on Artificial Intelligence
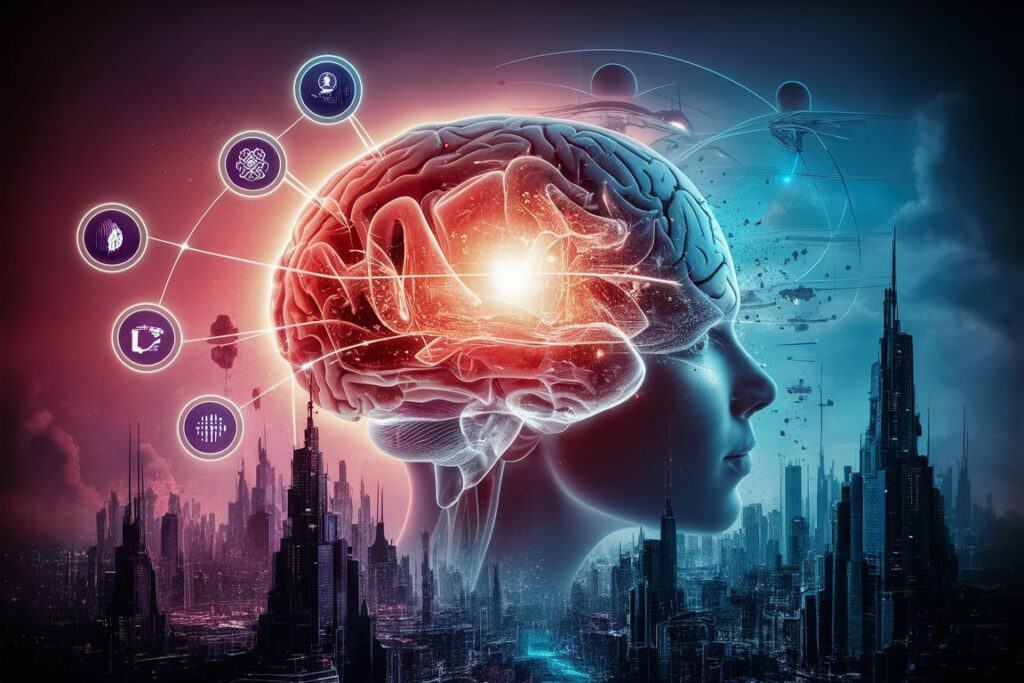
Some people confuse AI with being the final outcome of humanities’ work, instead of a tool which we can use to amplify our own ability and potential.
People fear that AI will take over jobs (which is true), but it will also create more jobs.
Previously unheard of jobs may become commonplace, such as algorithm auditors, human-machine interaction consultants, autonomous vehicle re-programmers (mechanics), and automated freight traffic supervisors.
The industrial revolution was a time when a lot of people lost their artisan jobs, which I am sure people feared at the time. However, instead of mass unemployment, more jobs were created. There was a need for assembly line workers, factory supervisors and more advanced human resources experts. This led to exponential growth of economies, as people could now use this extra time & efficiency to focus on more productive tasks, which inevitably opened the floodgates to more innovation, possibly leading to the introduction of computing & artificial intelligence itself. New developments pave the path for further progression.
A similar analogy of the industrial revolution can be applied to the impact of progressions in AI. Where the former streamlined our ability to make physical things, AI will allow us to solve more complex conceptual problems, in addition to fully automating the simpler physical tasks (consequential advancement of industrialisation). Not only will it improve our general societal output, but it also has the ability to improve our own intelligence and understanding of the world.
Despite how it may seem, our brains are just biological neural network machines, with billions of neurons interacting and communicating with each other through electrical synaptic pulses. This gives us the ability to think, speak, move, plan and act; independently. How these neural networks transpire into consciousness is still unknown – maybe our interaction with AI will give us the necessary pieces to solve this everlasting puzzle. Nature “programmed” this biological intelligence into us over millions of years. There are immense opportunities for us to learn from our man-made, silicon counterparts.
As we live in the present, with the immense power of hindsight at our disposal, we like to think that we are as advanced as we will get. Foresight, however, is not as powerful. It is hard to imagine how the world will look a couple of centuries from now, there are just too many variables to accurately predict the future, and with the ever-increasing pace of progression, historical experience is not representative of the future. A few hundred years from now, people may look back on us in the same way as we now look back on people from the medieval era.
Connection to Actuarial Work
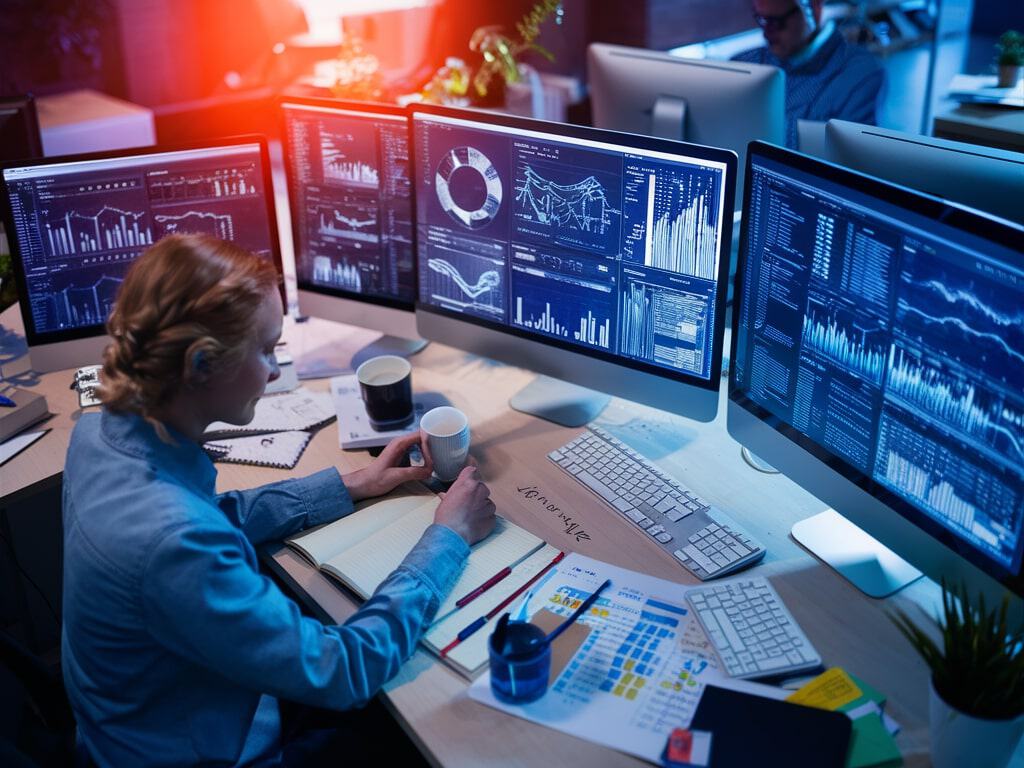
Actuaries are responsible for a multitude of complex tasks, which include not only building predictive models, but also qualitatively interpreting and building a narrative/story around their results, which machines still have difficulties doing. Despite what people may believe, the key feature that is still missing from machines is creativity.
A machine can answer a well-defined question better than any human can, but the real power comes from asking the right question in the first place.
Neural network and deep learning models are something that isn’t widely adopted within the actuarial industry yet (a “deep” model consists of a neural network model with more than one “hidden layer”). One key benefit of using such models is that they can find complex features from within the data, without the need for human intervention. Currently, there is a lot of value placed on an actuary’s professional judgement for the purpose of formulating models which is supported by, for example, speaking to underwriters and claims handlers and then using this knowledge to adjust various model parameters.
Using neural network models to learn complex features from data could remove this need for human intervention, or professional judgement, to achieve an actuarial result. This would allow actuaries to focus on interpreting the results of these models, instead spending time to achieve an accurate model by manually adjusting parameters using the industry knowledge they acquired for a specific line of business.
To give a practical example of how AI can be applied in the actuarial world, let’s look at the insight of Mario V. Wüthrich, and his implementation of neural networks to chain-ladder reserving methods.
Firstly, to approach any modelling tasks where you wish to apply an artificial intelligence model, you need to think about how to correctly phrase the “question” to our problem.
As long as a problem can be expressed in a differentiable way (i.e., in the form of a regression), then a neural network approach could be applied. With a bit of thought, actuarial problems such as estimating the loss reserves using a basic Mack Chain-Ladder Model can be interpreted as a regression with one independent factor, the cumulative claims at time t-1, which is adjusted by our parameter, the loss development factor, to produce cumulative claims at time t. This model can then be adjusted by applying a non-linear neural network regression to each development period to estimate a more accurate loss development factor.
This approach overcomes the assumption that portfolios are homogenous enough so that reserving methods, such as the one mentioned above, can be applied to each portfolio independently. The benefits of applying a neural network approach to loss reserving come from the fact that the neural network can make use of a multivariate approach, extending the feature space to include components such as line of business, claims code and accident quarter; and then fit a neural network to the expanded function. Once this function is estimated, it can be used to derive the loss development factors at this higher level of granularity, which you can then implement into your regular chain-ladder model to project a reserve.
This allows the network, once applied, to learn not only how each line of business develops independently, but also how these lines interact with each other on a grander scale, which may possibly allow us to see if there are any potential synergies between differing portfolios.
At present, for example, classes like motor and property are generally reserved independently of each other, as current reserving methods themselves do not allow for interactions between these portfolios. So, instead of a reserving actuary manually modifying the underlying assumptions of a portfolio which has been recently influenced by events from another line of business, a neural network approach could do this automatically at a higher level of abstraction. This could provide greater predictability to the reserving departments, potentially leading to lower losses and greater profitability to the insurance company. In addition, it could provide greater insight for other business departments, such as developing & improving their pricing algorithms for more efficient portfolio optimization.
Only relatively recent breakthroughs in the field of AI are allowing us to think about how to generalise these models to our tasks. There is a lot of potential there not only for improving existing processes and methodologies, but also for creating novel approaches, which may be revealed to us with further research within this field.
Professional Judgement—a Quantitative Result is Not Enough?
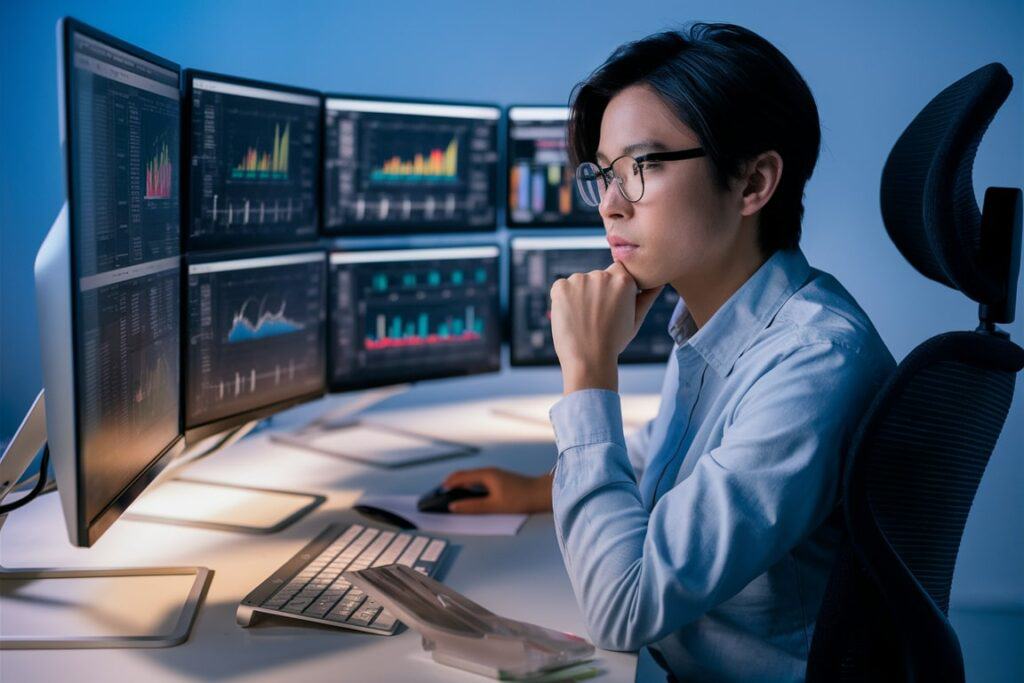
There’s just something about human intuition, which is the product of confidence and experience, that provides answers in the most unexpected of circumstances. Intuition is where the power of “professional judgement” comes from, and it allows us to solve difficult problems by incorporating both qualitative and quantitative aspects into our decision making.
Professional judgement is a powerful tool for actuaries.
There are a multitude of specialty risks which have very sporadic historical data for which this actuarial intuition is priceless. To give a couple of examples, natural catastrophe portfolio losses which include earthquake cover are notoriously hard to predict—earthquakes can happen anytime, anywhere without prior warning, and their severity on an insurers’ P&L is highly dependent on where it lands (e.g., 1994 Northridge Earthquake caused $15.3 billion in insured damages).
Another example would be political risk & terrorism portfolios, such portfolios may carry risk factors which cannot be accurately predicted using historical data—both the frequency and severity of these events can be truly unpredictable (e.g., before 11 September 2001 attack on the WTC no-one could’ve predicted the damage a terrorism act could do to the insurance industry, some claims—which total to approximately $47 billion -are still not fully run-off to this day, and this is considered one of the largest insured losses of all time).
When dealing with such portfolios, estimation of the quantitative ultimate losses is only a single factor of consideration, and decision to undertake such risks depend not only on the final predicted loss ultimate, but also on factors such as how these estimated losses would tie into the solvency requirements of the company, how they might affect reinsurance purchase decisions, and what are the general risk tolerance sentiments of the business. AI is still a very long way away from being capable of tackling such, sometimes undefined, complexity.
Another aspect to actuarial work, which may arguably be more important than what I mentioned above, is interpreting the key results. The overall result is nothing but digits & charts on a screen and can be meaningless to an untrained eye. Actuaries have the power to contextualise the problem, explaining what the result means to the business, and how the result feeds into other results. The meaning of a result can be highly dependent on the context at hand. For example, a start-up Insurtech may not look at the impact of a reserving portfolio in the same way that an incumbent insurer would. In both scenarios it may be proportionally the same number, but what it means to the company can be completely different.
This is what actuaries are particularly good at; seeing the bigger picture and how everything fits together at a higher level. And this is what matters most.
What Should Actuaries Do?
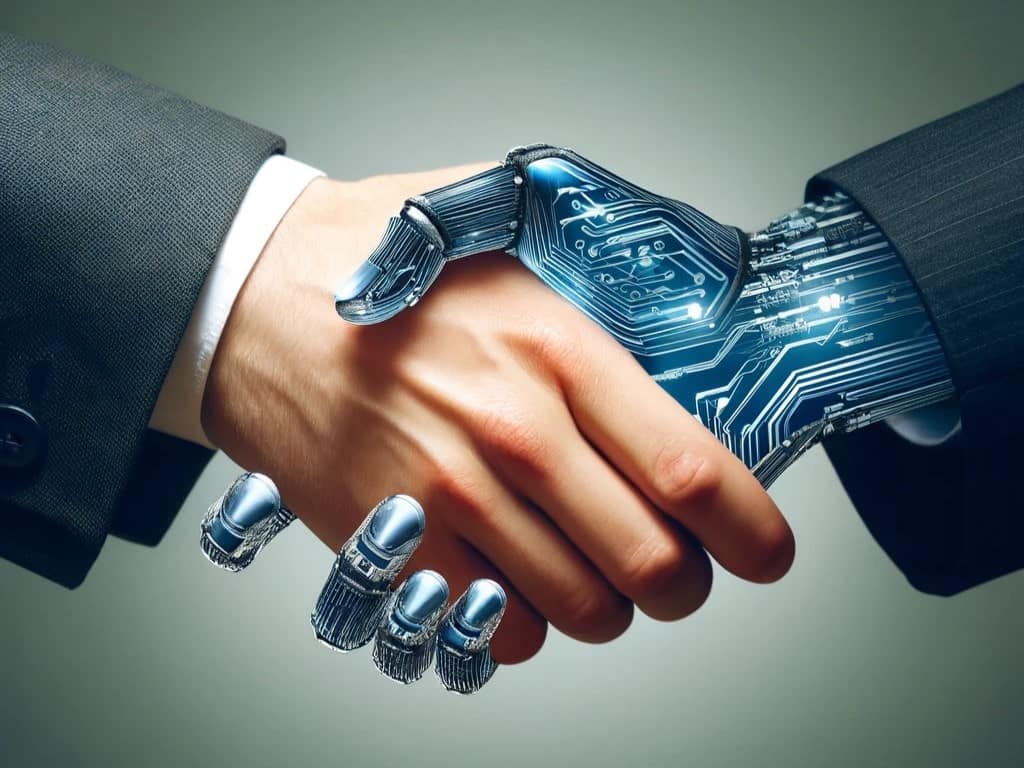
We need to leverage the power of AI to continue propelling our own capabilities at this rapidly increasing pace. If we brush it off as something inconsequential, we are at risk of dampening our own personal and professional development. There is a lot we can learn from artificial intelligence and it may allow us to see solutions to our problems from previously unknown perspectives (e.g., reserving example mentioned above).
It is much better for actuaries to adapt to this new world by learning how to implement various AI techniques into their work, instead of trying to fight it holding on to the dying status quo.
For actuaries, the power in their work can only be amplified with the use of AI.
The first step to understanding how to implement such models is to learn the linguistics of the machine. Actuaries need to understand how to communicate with computers, which means learning and improving their programming skills. Learning a language like Python may seem daunting, but this language is so versatile that it may provide limitless opportunities down the line, even paving the path to understanding more specialised languages such as R, SQL, and C++. Taking the first step is always the hardest, but sometimes it is the most important.
Concluding Thoughts
For now, I believe actuaries shouldn’t worry about AI taking over our actuarial jobs, not until Artificial General Intelligence (“AGI”) has reached further developments.
In some distant future, however, when AGI has reached more fundamental breakthroughs, allowing machines to “think” for themselves, this is when we should start worrying about AGI taking our jobs. But realistically, by that stage if they’re doing actuarial work for us, that means the rest of society may lose their respective jobs as well.
Maybe in this utopian future we will all be out of jobs allowing us to spend our days for the purposes of leisure, creative expressions and athletic feats.
But for now, worrying about whether or not AI will take over anyone’s job is a purposeless act.
Instead, we should learn to make use of the immense power and potential of AI to improve and increase our actuarial (and societal) output.